Cardiovascular Genomics and Systems Biology
Is Heart Disease Genetic?
Researchers at the UCLA Cardiovascular Theme are investigating and contributing to our understanding of the genetic basis of heart disease.
Heart disease is the epidemic of the 21st century with almost 20 million people globally and more than 6 million people in the United States carrying the diagnosis. More than 600,000 individuals are annually diagnosed with heart disease in the US alone—and once a diagnosis is made, the 5-year survival rate is close to 50% despite optimal medical therapy. Despite major advances in medical technology and science, little is known about genes that can affect survival or denote accelerated progression of heart disease. The UCLA Cardiovascular Theme uses comprehensive genomics, systems biology and multi-omics approaches to solve such questions.
Finding Genes Involved in Heart Disease
One example of a condition caused by genetic mutations that run in families is hypertrophic cardiomyopathy. This is the disorder often associated with sudden death in young athletes.
- Hypertrophic cardiomyopathy affects up to 500,000 people in the United States.
- While some family members have a mild form of the disorder, others can have a severe outcome.
- The chance of inheriting a mutation is 50/50.
- Up to 70 genes have been implicated in this disorder.
Dr. Jessica Wang, Assistant Professor of Medicine in the Division of Cardiology, leads an effort to understand how variation in the DNA sequence causes heart disease.
- Inherited Cardiovascular Disease Registry: Dr. Wang is leading an effort to investigate the devastating cardiovascular diseases that often strike early in life and that run in families and is partnering with the Institute for Precision Health at UCLA. Dr. Wang is performing “exome” sequencing on many such patients and is correlating genetic information with electronic health records to identify genes which could be more informative of patient outcomes or prognosis “This effort is only possible because of the crosstalk we have with all the other disciplines on campus,” she says.
- Finding effective therapies: Dr. Jessica Wang hopes that systematic collection of genetic information coupled with the electronic health record as pioneered by the Institute for Precision Health at UCLA will provide unprecedented opportunities for guiding care of individuals with heart disease.
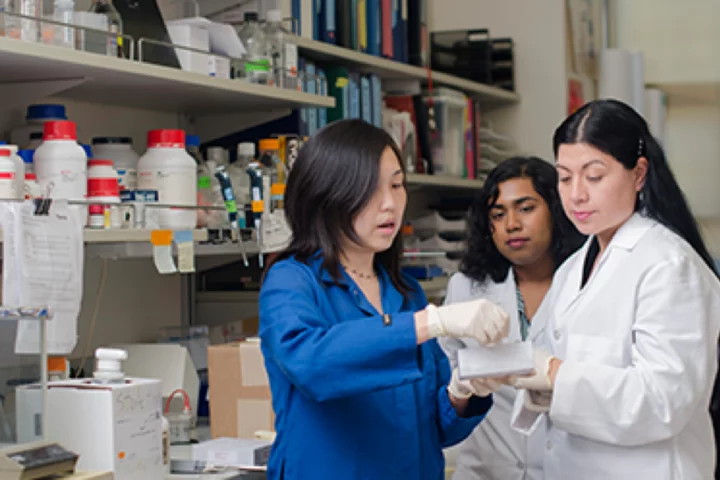
Cardiovascular Systems Biology
The laboratory of Professor Jake Lusis in the departments of Cardiology and Human Genetics uses a multi-omic approach to identify key genetic pathways that affect heart disease. The laboratory uses the combination of genetics, molecular biology, and informatics to investigate pathways underlying common cardiovascular and metabolic disorders. They exploit natural genetic variation among inbred strains of mice or human populations to identify novel targets and formulate hypotheses, and perform validation using experimental perturbations in mice.
The lab has taken advantage of high-throughput biologic technologies, such as transcriptomics, metabolomics, and proteomics, to help develop new approaches to understand complex traits. They have shown that when integrated with natural genetic variation, such multi-omics analyses can be used to model biologic pathways and uncover regulatory mechanisms—an approach known as “systems genetics”.
During the past 10 years, they have developed a unique mapping approach in mice that is analogous to genome-wide association studies in humans. This approach utilizes a well-characterized population of 100 inbred strains of mice, termed the Hybrid Mouse Diversity Panel (HMDP). A major advantage of this approach is that the resolution is much better than with traditional genetic crosses. It also facilitates studies of gene-by-environment interactions, which are very difficult to address in human populations, and it has important advantages for systems-based approaches which require analysis of relevant tissues.
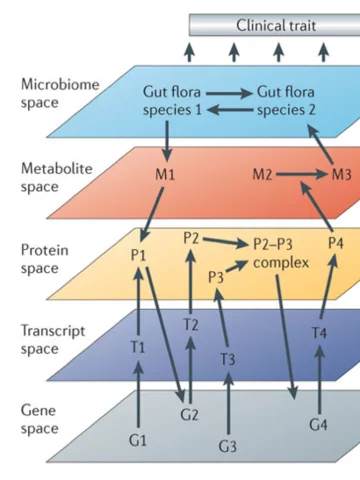